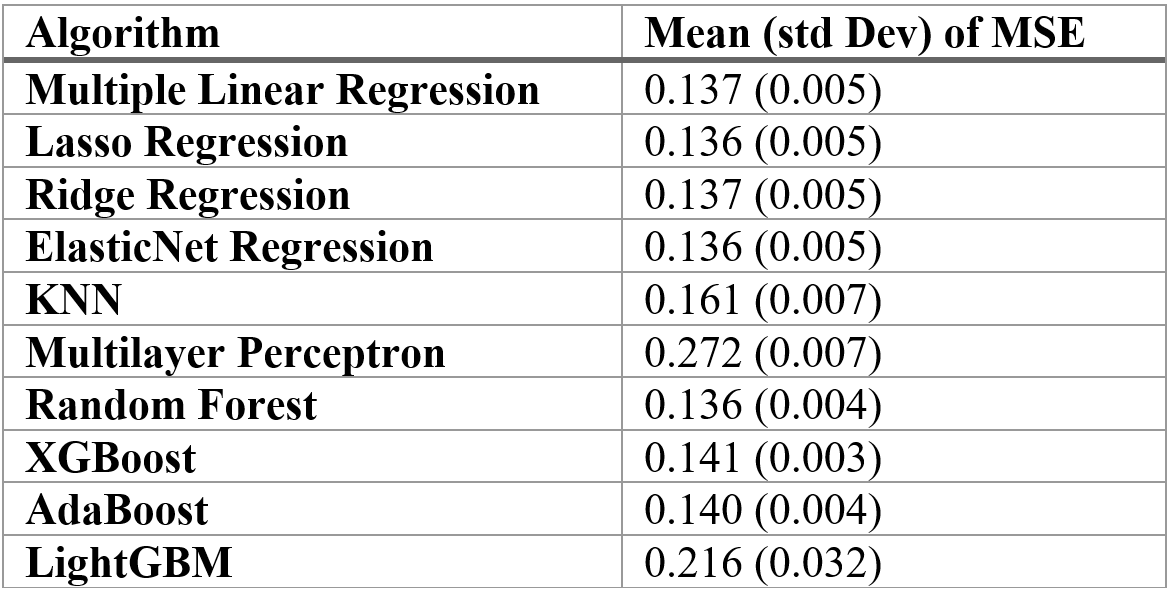
Stroke Prediction & Analysis
This project applies machine learning techniques to address the critical challenge of stroke prediction, integrating theoretical concepts with practical healthcare applications. Using data from the Behavioral Risk Factor Surveillance System (BRFSS), the study leverages Random Forest for feature selection, validated by medical knowledge, to identify key predictors of stroke. The XGBoost model, chosen for its balance of accuracy and robustness, achieved a reliable prediction rate of 4.1% on the test set, closely aligning with real-world onset rates. Extending the approach to heart disease patients, the model predicted a 14% stroke onset rate with an F1 score of 0.84, demonstrating high sensitivity. By combining feature engineering, robust machine learning, and healthcare insights, this research tackles practical medical challenges with cutting-edge computational methods.